General Finance
Dec 18, 2024
•
5 min read
Identifying AI Opportunities in Financial Services: Unlocking Efficiency, Growth, and Competitive Edge
Overview
In today’s rapidly evolving financial services industry, firms face increasing pressure to adopt AI solutions that drive operational efficiency, reduce costs, and improve customer experience. While headlines spotlight AI tools like chatbots, image generators, and coding assistants, their relevance to the complex needs of financial institutions may seem limited. However, the real power of AI lies in solutions tailored to address the unique challenges of financial services—from portfolio optimization to risk mitigation.
When approached strategically, AI empowers firms to streamline processes, uncover insights, and achieve scalable growth. With the global financial services AI market projected to reach $64 billion by 2030, now is the time to align your AI initiatives with your business goals.
Defining the Problem
It’s tempting to view AI as an off-the-shelf product that can solve all problems. While standardized AI tools provide some benefits, they rarely deliver a competitive edge. To maximize impact, financial institutions need to take a thoughtful problem-first approach - tailoring AI solutions to their specific data environments, workflows, and strategic objectives.
Framing the Problem: 3 Things to Consider
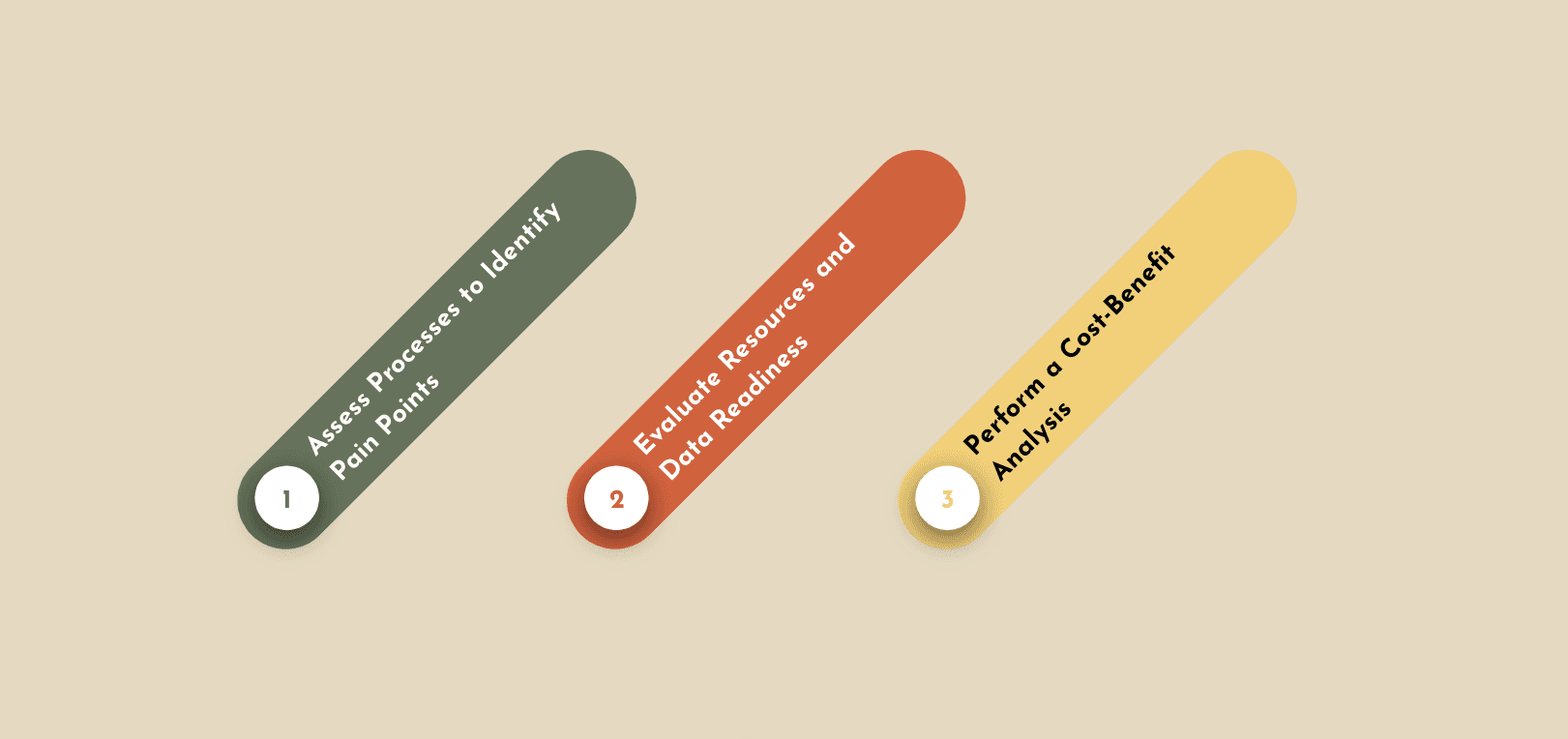
Assess Processes to Identify Pain Points: The financial services industry is data-heavy, with many workflows relying on unstructured or semi-structured data. By collaborating with your team, you can identify inefficiencies, processes that are error-prone and manual workflows that tend to slow down your operations. Ask yourself, which workflows are redundant or tie up your resources? Focus on the data that’s unique to your business and identify bottlenecks within it.
Processes like credit underwriting, loan approvals, or regulatory reporting that involve repetitive data analysis and human intervention.
Evaluate Resources and Data Readiness: AI success depends on data readiness. Unfortunately, 80% of enterprise data is unstructured, presenting challenges in integration and usability (Gartner). However with modern AI tools, you can transform this data into actionable insights.
Take a wealth management firm, for example. By converting unstructured client communications, performance reports, and regulatory filings into a unified, analyzable resource, the firm can deliver personalized portfolio recommendations with greater accuracy and efficiency.
Perform a Cost-Benefit Analysis: This will be both a quantitative and qualitative exercise. The quantitative aspect is more straightforward. Quantitatively, evaluate how AI can reduce costs, improve productivity, or enhance accuracy. On the qualitative side, consider how these improvements free employees for higher-value tasks, enhance client satisfaction, or reduce operational friction. Ask yourself if greater efficiency will create an improved customer experience, leading to less friction and increased ROI (e.g. revenue, new users, etc.).
Your AI Advantage Starts with Your Data
AI thrives on data, and financial institutions are uniquely positioned with vast stores of it across three primary categories. However, not all data is created equal, and leveraging it effectively is more complex than it may seem. Let’s explore the different types of data that financial institutions commonly encounter.
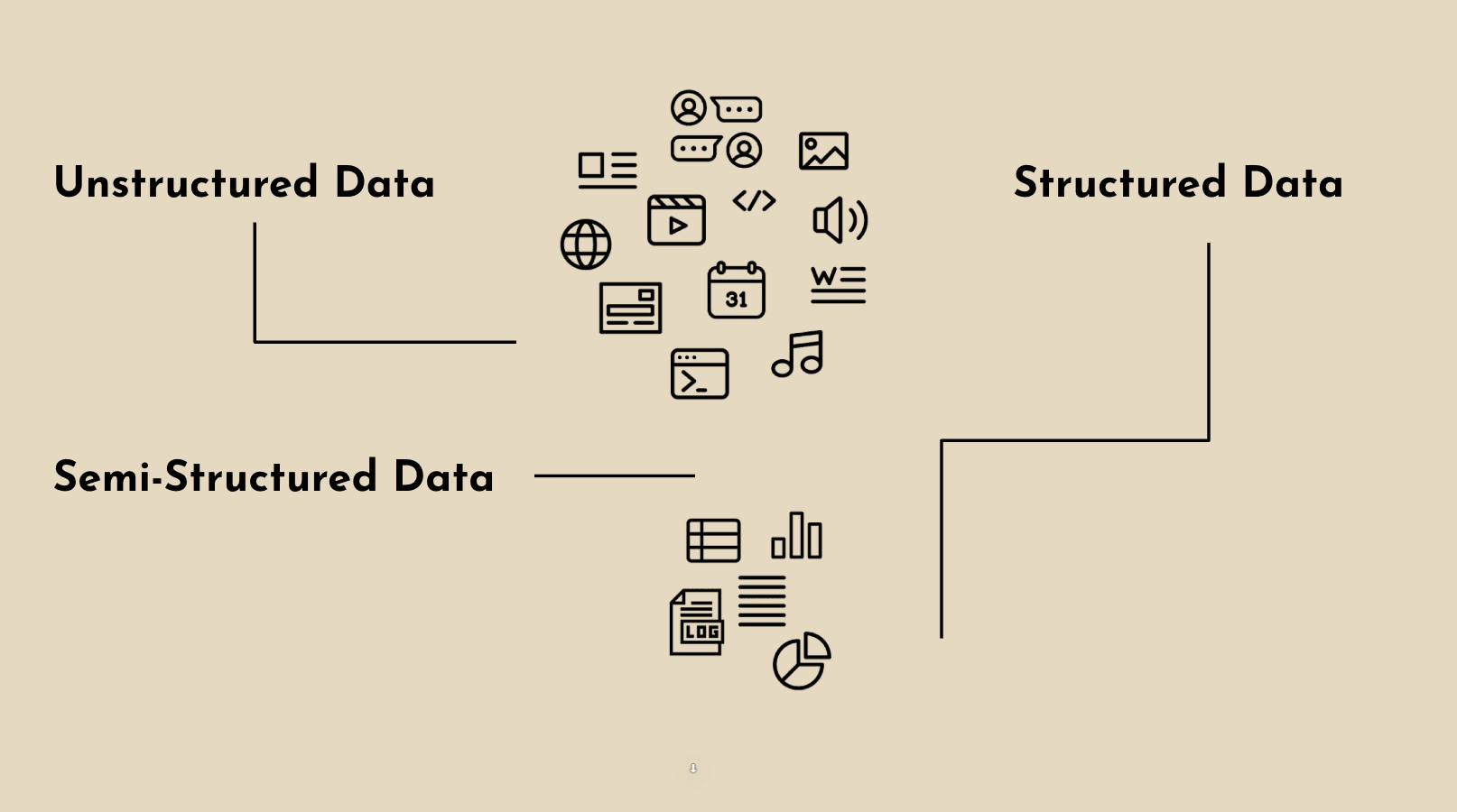
Unstructured Data: This is data without defined formats, labels, or organization within a database—a common challenge for financial institutions. It includes client communications, regulatory filings, investment research reports, legal documents, loan applications, or customer feedback. Unstructured data often resides in text-heavy formats like PDFs, emails, or images, making it difficult to analyze and integrate into workflows. Yet, it holds immense potential for generating actionable insights.
What are some real-world examples? AI can analyze regulatory filings and market reports to identify compliance risks, evaluate portfolio exposure, or uncover emerging investment opportunities. In wealth management, it can derive insights from unstructured client communications to provide tailored portfolio recommendations.
Structured Data: Structured data is well-organized, labeled, and fits neatly within databases—making it easier to process and integrate into workflows. This includes financial records, transaction histories, portfolio data, and market feeds. While structured data receives the bulk of attention, its value multiplies when combined with unstructured data for deeper context.
Most investment manager are already leveraging structured trade and transaction records. However if you can pair that with unstructured market news, investment managers can enhance their ability to predict market movements and identify alpha-generating opportunities.
Semi-Structured Data: Semi-structured data falls between unstructured and structured data. It has some level of organization but lacks a standardized structure that can be easily mapped to existing systems. Examples in financial services include invoices, loan documentation, KYC (Know Your Customer) records, and performance reports. Semi-structured data often requires additional processing to unlock its full value.
By leveraging AI, you can extract key metrics from semi-structured data like loan agreements and integrate them with structured credit data to streamline underwriting processes, reduce loan approval times and improve risk assessments.
The Impact
Although benefit can be realized relatively quick, AI should be viewed as a long-term investment that requires patience and phased implementation to achieve its full benefit. Be mindful of your timeline and break your goals into the following categories:
Short-Term Gains: Make no mistake, properly leveraging your data provides immediate impact. Quick wins from automating mundane, discrete, or transactional tasks help build momentum in your overall process improvement strategy. According to McKinsey's latest research, AI can reduce loan approval times by up to 30% through automated credit scoring and document verification. While this may not represent the most groundbreaking use of AI, automation remains one of the most practical and impactful real-world applications we’ve seen.
Medium-Term Improvements: Other processes might require additional time to exhibit payoff. Front-line defenses such as audit monitoring, fraud detection, or customer service improvements might not produce value for a few weeks. Benefit will be realized as efficiency increases make your workforce more productive and less error prone. Take fraud detection for example. AI can help financial institutions reduce false positives by 20-30%, saving millions in operational costs and improving accuracy.
Long-Term Transformation: Areas such as product automation, predictive maintenance, or advanced risk management systems provide the opportunity to transform your business on a holistic level. As you gain control of your data, competitive advantages will distance you from late adopters.
Payoff Comes in All Shapes and Sizes
AI has practical, real-world applications across all segments within financial services. Although it’s best to focus on your areas of expertise, benefits can be found across every aspect of your business. Here are just a few examples from some of our customers that has successfully deployed AI-powered solutions.
Conclusion
The key to successful AI adoption lies in identifying the right opportunities. Just as you wouldn’t design a house based on the tools you have, don’t limit process improvement to only off the shelf products. Design the house, then acquire the appropriate tools for the job. By carefully examining workflows and understanding their data environment, enterprises can sharpen their competitive edge. At Gradient, we help businesses discover and quickly implement tailored AI-driven solutions. Building on short-term improvements while planning for long-term transformative changes, Gradient’s Finance Reasoning Platform provides an effective, cost-efficient method to put your company at the leading edge of AI implementation. Check out our deep dive into data reasoning or answer these 5 simple questions to see if data reasoning is right for your business.
Share